Aplicación de técnicas de ML/IA y Edge Computing para la Movilidad Sostenible: Perspectivas del Proyecto SUCCESS-6G
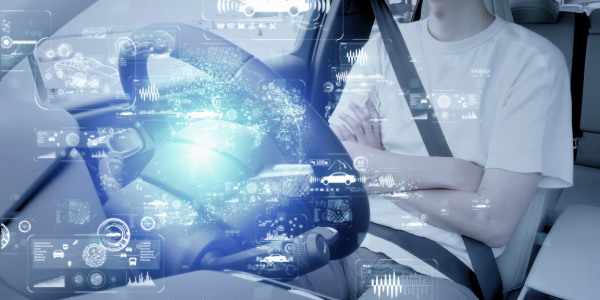
Several key technological advancements in the ability to transmit, process and analyse vast amounts of vehicular data are deeply transforming and opening new possibilities within the domain of autonomous and connected vehicles.
5G and emerging 6G networks enable vast data acquisition and scalable and flexible system deployments, allowing for connectivity and vehicle operations to function effectively. Artificial intelligence (AI) has been increasingly applied to the condition monitoring of vehicular equipment, aiming to improve safety, reduce costs, and optimize maintenance strategies. MEC (Mobile Edge Computing) enables faster processing and local decision-making by keeping data close to its source.
SUCCESS-6G project, led by CTTC and with the collaboration of several industry partners, takes on the challenge of integrating these technologies to facilitate the identification of vehicular anomalies and optimize operations through two use cases: i) real-time vehicle condition monitoring and fault provisioning and; ii) over-the-air vehicular software updates.
Within this framework Optare has contributed to:
- extract knowledge from car data streams for fault diagnosis
- develop AI-driven intrusion detection models to secure V2X communications
- explore decentralized model training using federated learning techniques
Key Innovations areas of SUCCESS-6G
Below is a detailed breakdown of the key innovation areas addressed by the SUCCESS-6G project, organized around technical capabilities, architectures, and applications in connected and secure vehicular environments. This approach spans from network infrastructure to the integration of advanced artificial intelligence technologies, service optimization, and real-time monitoring for autonomous and connected vehicles.
1. Private 5G Networks and Edge Computing
- Private 5G Network: Deployment of dedicated private 5G infrastructure designed specifically for smart mobility applications, particularly for autonomous vehicles. This isolated and secure network provides higher reliability and quality of service, especially in high-demand and mobile environments.
- Edge Computing: Deployment of edge nodes that process data locally, reducing latency and improving efficiency in critical services, such as handover processes (connection transfers between nodes). This infrastructure allows vehicles and connected devices to operate more quickly and efficiently in dynamic environments.
2. Advanced Real-Time Monitoring
- Performance Monitoring: Advanced tools to measure real-time performance, including response times and network stability during handover processes between 5G nodes. This monitoring helps assess network behavior during vehicle mobility, identifying fluctuations and ensuring continuous service.
- Observability Infrastructure: Implementation of time-series databases as a centralized platform for storing and analyzing performance metrics. This enables detailed and predictive analysis of potential network failures, aiming to improve operational resilience and stability.
- Real-time Data Visualization: Real-time visualization of metrics and performance statistics through histograms and other charts, making data-driven decisions easier to optimize performance during real-world test scenarios, such as those conducted at the Castellolí circuit.
3. Machine Learning (ML) & AI Technologies
- Machine Learning Integration: Integration of advanced Machine Learning and Artificial Intelligence techniques to optimize anomaly detection and predictive maintenance. Use of AI-based inference models to detect malicious behaviors and improve operational efficiency in vehicular environments.
- Real-Time Anomaly Detection: Implementation of ML/AI systems to detect faults in real time, helping identify vehicular behavior anomalies, sensor malfunctions, and security vulnerabilities in vehicle communications.
- Transparency & Explainability: Use of tools like SHAP to ensure model interpretability, which is essential in vehicular safety and diagnostics, providing clear explanations of how decisions are made within the system.
4. Dynamic Service Orchestration
- Orchestrator: Manages the dynamic migration of services between edge nodes, adjusting network resources according to vehicle mobility conditions. This ensures service continuity as vehicles move between different 5G nodes.
- Scalability: The orchestrator allows for dynamic scaling of network resources, ensuring that the network can adapt to real-time demand changes.
5. Intelligent Vehicle Condition Monitoring Under 5G
- Monitoring Objective: Evaluate the inference system’s performance in real-time during vehicular mobility in a 5G environment, paying particular attention to response times and system stability during handovers between 5G nodes (Edge_1 and Edge_2).
- Technological Milestones: Precise measurement of post-handover response times and monitoring of fluctuations during network transfers. Components like kServe, Mediator, InfluxDB2, and Prometheus were integrated for continuous observability of performance metrics.
6. Security Framework with AI-Based Malicious Behavior Detection
- Security Objective:
- Monitoring vehicle data and real-time detection of malicious behaviors using a Deep Reinforcement Learning (DRL)-based detection model, specifically designed to address common attacks like data replay and Denial-of-Service (DoS).
- Technological Milestones:
- Use of the SUPERCOM platform for simulating OBUs and RSUs, integrating the AI-based inference model to detect security faults.
- Evaluation of model security metrics such as Accuracy, Precision, Recall, F1-Score, with additional metrics like MTTD (Mean Time To Detect) and MTTR (Mean Time To Respond) to assess attack response effectiveness.
7. End-to-End ML Pipeline for Anomaly Detection
- ML Pipeline Design:
- Design and implementation of a comprehensive machine learning pipeline, from data collection to optimization and production deployment. This pipeline effectively detects anomalies and is scalable for use in connected vehicles in real-world scenarios.
- Technological Milestones:
- Deployment of a central model based on LightGBM optimized with Optuna for hyperparameter selection, ensuring high performance.
- Explainability analysis using SHAP to provide transparency in the model’s decisions, ensuring user trust in the diagnostic system.
8. Advanced Anomaly Injection and Evaluation
- Anomaly Injection: Controlled injection of sparse and collective anomalies into real datasets to evaluate the system’s robustness to different types of faults, from sporadic faults to persistent issues.
- Evaluation Results: High precision in detecting persistent faults, but a need to improve model sensitivity to sporadic faults, where recall is lower.
9. Real-World Deployment and Telemetry Monitoring
- Production Deployment: Validation of the ML system in a real production environment, using InfluxDB for data traceability and continuous monitoring of model inferences under real-world driving conditions.
- Technological Milestones: Continuous evaluation of performance metrics in dynamic, real-world environments, such as the Castellolí circuit, with detailed tracking of telemetry data generated by connected vehicles.
Conclusions
The integration of advanced service orchestration solutions, edge computing, and AI-driven real-time monitoring promises seamless and secure user experience for applications like autonomous or connected mobility enabled by 5G/6G networks.
By deploying and validating a robust, secure and computationally efficient approach for realizing new vehicular services, the research conducted under SUCCESS-6G project contributes to address mobility challenges and shape tomorrow’s transportation systems.
Acknowledgments
SUCCESS-6G has received funding by the Spanish Ministry for Digital Transformation and Civil Service, and co-funded by the European Union – NextGenerationEU program via the Recovery and Resilience Facility (references TSI-063000-2021-39/40/41). The project consortium comprises of the Centre Tecnològic Telecomunicacions de Catalunya (CTTC), Optare Solutions, NearBy Computing, Idneo and Cellnex, with the goal of advancing smarter and more sustainable connectivity in the field of mobility and autonomous vehicles.